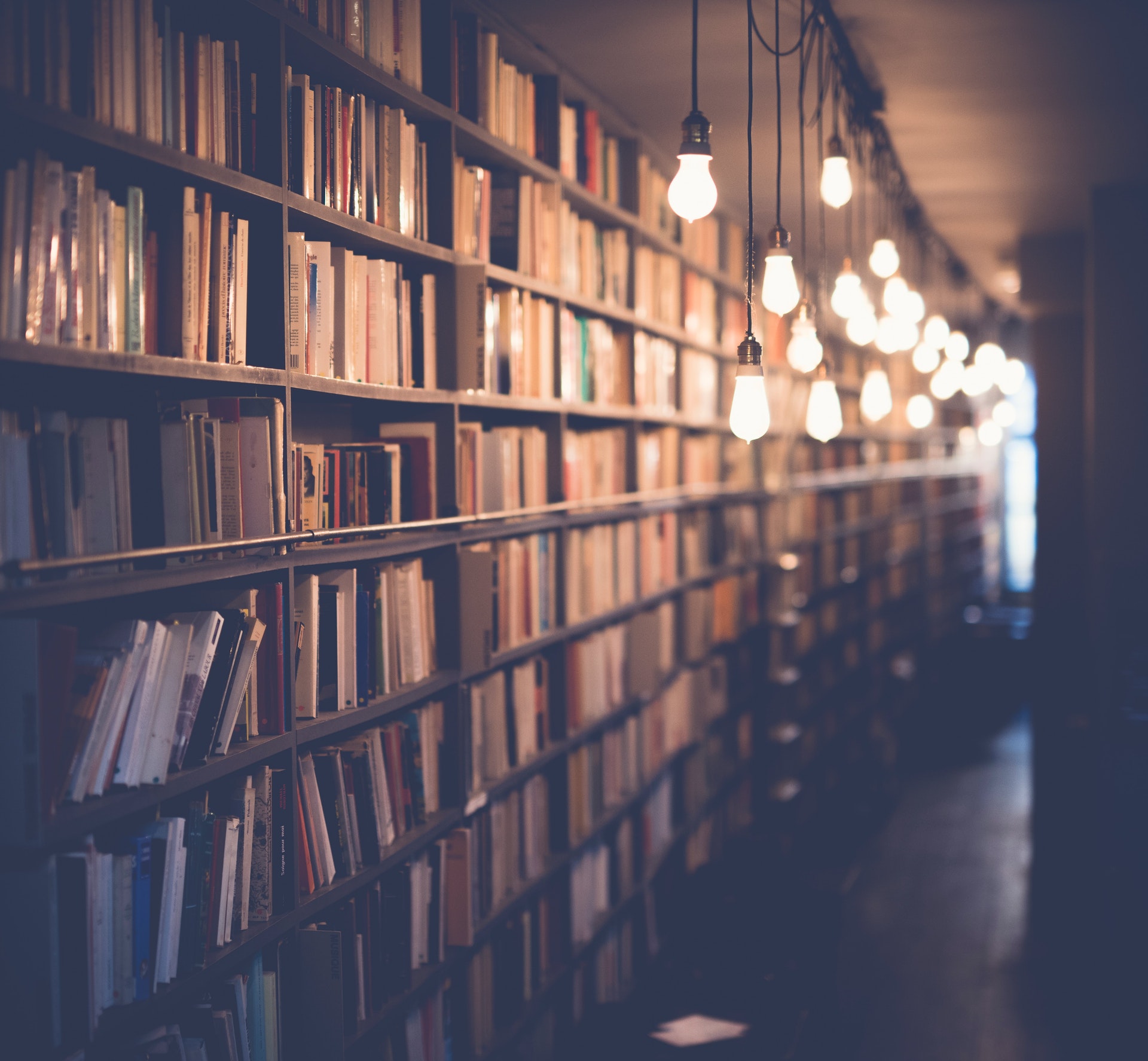
The table that shows no correlation is the one that is blank. There is no information in it to suggest any relationship between the variables. This could be because the data is too scattered, the variables are not actually related, or there could be some other reason. It's impossible to say for certain without more information.
What is the table showing?
The table below shows the different types of housework done by men and women in the US.
It's no surprise that women generally do more housework than men. In fact, according to the table, women do an average of nearly twice as much housework as men. The most common type of housework done by women is cleaning, which includes tasks like dusting, vacuuming, and mopping. Women also do more laundry than men, and are more likely to cook meals from scratch. Men, on the other hand, are more likely to do yard work and home repairs.
So what does this all mean? Well, it's likely that the division of labor in most households is still fairly traditional, with women taking on the majority of the domestic work. This is likely due to the fact that women are still more likely to be stay-at-home moms than men, and even working mothers are often the ones who take on the majority of domestic duties. However, it's important to note that the gap between men and women's housework participation is slowly but surely closing. In fact, the percentage of men who do housework has increased steadily over the past few decades. So, even though the table shows that women still do the vast majority of housework, things are slowly changing.
For your interest: Research Table Work
Why is there no correlation?
There is no correlation because there is no relationship between the two variables. They are not associated with each other. There is no pattern or order to their arrangement. They exist independently of each other.
What does this mean for the data?
The phrase "What does this mean for the data?" is often used in discussions about data interpretation and analysis. It is a question that highlights the importance of understanding the implications of data results.
When data is collected, it is important to think about what the data will be used for. Will it be used to make decisions about policy or allocations of resources? Will it be used to better understand a phenomenon? Will it be used to evaluate the effectiveness of a program? The answer to this question will help determine how the data should be collected and analyzed.
Data interpretation is the process of turning data into information that can be used to make decisions. This process can be complicated, and it is important to consider all of the possible implications of the data before making any decisions. When data is interpreted, it is important to ask questions such as "What does this mean for the data?" in order to ensure that all of the possible implications are considered.
Consider reading: What Does a Correlation of -1 Mean?
What could be the reason for this?
There can be many reasons for this. Maybe the person is going through a tough time and needs someone to talk to. Maybe they feel like they don't have anyone to talk to. Maybe they're just feeling lonely. Maybe they need someone to help them with something. Maybe they need a friend.
What does this tell us about the relationship between the two variables?
There are a few things that we can gleam from this data about the relationship between the two variables. First, we can see that there is a clear linear relationship between the two variables. As one variable increases, so does the other. Additionally, we can see that the relationship is relatively strong, as the points are close to the line of best fit. This tells us that the two variables are likely related in some way, and that changes in one are likely to cause changes in the other. Finally, we can see that the relationship is not perfect, as there are some points that deviate from the line. This could be due to other factors that are not accounted for in this data, or it could simply be noise. Overall, this data tells us that there is a strong linear relationship between the two variables, and that changes in one are likely to cause changes in the other.
What other information would we need to know in order to understand this better?
In order to understand a situation better, we often need more information than what is initially given. More details can help to provide context and clarity, helping us to better understand the situation.
For example, imagine you are told that there was a car accident. In order to understand the situation better, you would likely want to know more details such as where the accident occurred, what time it happened, how many cars were involved, and whether anyone was injured.
Similarly, if you are told that a company is planning to lay off workers, you would likely want to know more information in order to understand the situation better. This information could include how many workers will be laid off, what positions they hold, where the layoffs will occur, and when they will take effect.
In many cases, the more information we have, the better we can understand a situation. However, there is such a thing as too much information, and it can be overwhelming or confusing. In these cases, it can be helpful to ask questions so that you can focus on the most important details.
Asking questions is a great way to get more information when you are trying to understand a situation better. However, it is important to ask questions that are specific and relevant to the situation. For example, if you are trying to understand a car accident, asking the police officer who responded to the scene for their opinion on what caused the accident would likely be more helpful than asking about the officer's favorite color.
In summary, we often need more information in order to understand a situation better. More details can provide context and clarity. It is important to ask questions that are specific and relevant to the situation. When we have more information, we can often understand a situation better.
Consider reading: Amortization Tables for Car Loans
What are the implications of this lack of correlation?
There are a few implications of the lack of correlation between the two variables. First, it could mean that the study was not well designed and that the variables were not chosen wisely. It could also mean that the study was not conducted properly and that the data was not collected accurately. Lastly, it could mean that the two variables are not actually related and that the study was unable to find a relationship between them. All of these implications are serious and could lead to the study being considered invalid.
What could we do to further investigate this?
When it comes to investigating something, there are a number of different approaches that one could take. Depending on the subject matter, some methods might be more effective than others. In general, however, there are a few things that could be done in order to further investigate this.
First, it would be beneficial to consult with experts in the field. This could provide insights that would otherwise be unavailable. Additionally, research could be conducted in order to gain a better understanding of the issue at hand. This could involve reading relevant literature, conducting interviews, or observing people or events.
Second, it would be helpful to develop a hypothesis or theories about what could be causing the problem. This could provide a direction for the investigation to take. Additionally, it could allow for the use of scientific methods to test these ideas.
Third, it would be useful to collect data. This could be done through surveys, experiments, or observations. This data could then be analyzed in order to look for patterns or trends. Additionally, it could be used to test hypotheses or theories that have been developed.
Fourth, it would be beneficial to discuss the findings with others. This could provide different perspectives and allow for debate. Additionally, it could lead to the development of new ideas.
Ultimately, there are a number of different things that could be done in order to further investigate this. By taking a variety of approaches, it should be possible to gain a better understanding of the issue at hand.
What are the potential consequences of this lack of correlation?
The potential consequences of the lack of correlation between educational attainment and income are manifold. Perhaps most obviously, individuals who have not attained a high level of education may find it more difficult to earn a high income. This is likely to lead to increased inequality between those with high and low incomes, as well as between those with high and low levels of education. Additionally, the lack of correlation between educational attainment and income may have negative consequences for social mobility. Individuals from lower-income backgrounds may find it more difficult to move up the socio-economic ladder if their educational attainment does not enable them to earn a higher income. This could lead to increased social stratification and reduced social mobility. Additionally, the lack of correlation between educational attainment and income may have negative implications for economic growth. If individuals with lower levels of education are less likely to earn high incomes, they may be less likely to reinvest their earnings back into the economy, leading to slower economic growth. Finally, the lack of correlation between educational attainment and income may also lead to political instability. If members of society feel that they are not being rewarded for their educational achievements, they may be more likely to engage in protests or other forms of civil unrest. In sum, the lack of correlation between educational attainment and income could have far-reaching and wide-ranging consequences, both for individuals and for society as a whole.
You might like: Correlation Coefficient
Frequently Asked Questions
How to test if there is no correlation between two tables?
For one-tail tests, multiply α by 2. If the calculated Pearson’s correlation coefficient is greater than the critical value from the table, then reject the null hypothesis that there is no correlation, i.e. the correlation coefficient is zero.
What does it mean if there is no correlation between variables?
There is no correlation between variables if the data points are scattered in a random pattern or form a curve. This could mean that there is no underlying relationship between the variables and that it's simply a coincidence.
How do you know if there is a correlation between data?
There are a few ways to determine if there is a correlation between data. One way is to plot the data points on a linear or straight line and see if there is a correlation. If there is, it means that the data points are associated with each other in a predictable way. Another way to examine correlation is to use a bubble chart. Bubble charts show how correlated data points are by their positions on the chart. When data points are clustered together, it indicates that there is a correlation between them.
What if the correlation coefficient of two variables is zero?
There is no linear relationship between the variables. However, this is only for a linear relationship. It is possible that the variables have a strong curvilinear relationship.
What if two variables have no correlation?
In this scenario, no clear pattern can be seen in the scatterplot because the correlation between the variables is zero.
Sources
- https://en.certificationanswers.com/google-analytics-certification-answers/you-have-ads-personalization-enabled-for-your-property-but-want-to-exclude-specific-events-what-does-this-mean-for-the-data-for-those-events/
- https://context.reverso.net/traduction/anglais-francais/table+showing
- https://corporatefinanceinstitute.com/resources/data-science/mean/
- https://www.statology.org/no-correlation-examples/
- https://jdmeducational.com/what-does-the-mean-tell-you-about-data-3-key-ideas/
- https://www.tableau.com/blog/what-does-data-mean-you-tell-us-two-words-60405
- https://globalizethis.org/which-table-shows-no-correlation/
- https://www.linguee.fr/anglais-francais/traduction/attached+is+a+table+showing.html
- https://sciencetopics.quest/trending-ask/which-table-shows-no-correlation/
- https://www.linguee.fr/anglais-francais/traduction/there+is+no+correlation.html
- https://www.simplilearn.com/what-is-data-article
- https://homeworkmarketonline.com/homeworks/detail/11457/which-table-shows-no-correlation/
- https://globalizethis.org/which-table-shows-a-negative-correlation/
- https://enya.day/mathematics/question-3342079.html
Featured Images: pexels.com