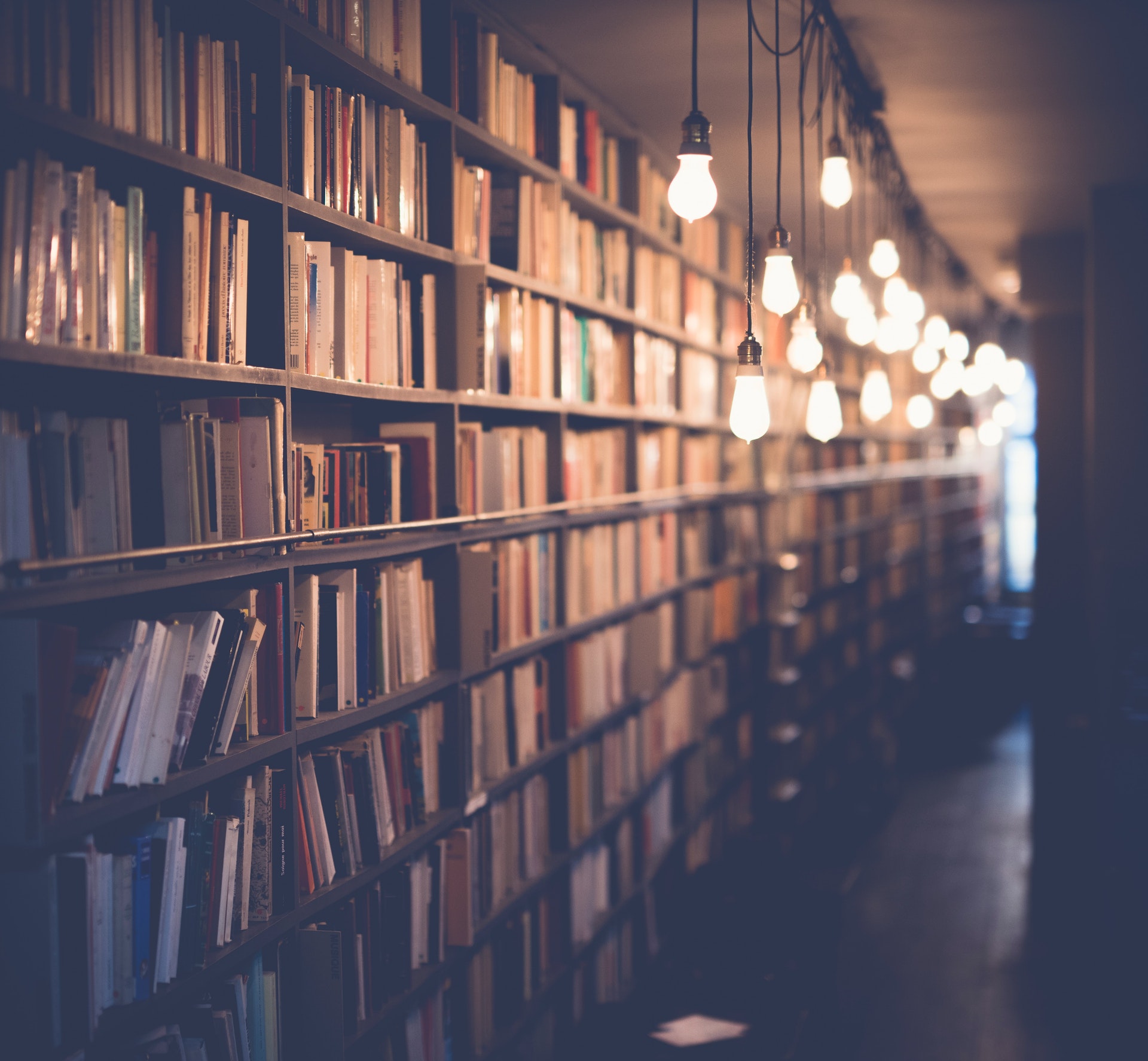
There are a variety of models which are characteristic of nonrational decision making. These models include the satisficing model, the expectancies model, and the linear programming model. Each of these models has its own shortcomings, but collectively they help to illustrate how nonrational decision making can be understood and analyzed.
The satisficing model is based on the idea that people make decisions by choosing the first option that meets their minimum requirements. This model is helpful in understanding how people make simple decisions, but it fails to explain how people make more complex decisions. The expectancies model is based on the idea that people consider the expected outcomes of their choices before making a decision. This model is helpful in understanding how people make decisions based on their goals and values, but it does not always accurately predict how people will behave. The linear programming model is based on the idea that people make decisions by optimizing a linear combination of constraints and objectives. This model is helpful in understanding how people make decisions when they have multiple conflicting goals, but it fails to take into account the human factor in decision making.
Collectively, these models help to illustrate how nonrational decision making can be understood and analyzed. They also highlight the shortcomings of each model and how those shortcomings can be addressed.
Readers also liked: Human Weaknesses Complicate Decision Making
What are the main features of nonrational models of decision making?
Nonrational models of decision making can be distinguished from rational models in a number of ways. First, nonrational models tend to emphasize the role of emotions and other non-cognitive factors in shaping decision making. Second, nonrational models de-emphasize or even ignore the role of deliberative reasoning in favor of more intuitive processes. Third, nonrational models often involve a greater degree of risk taking and are less concerned with the idea of maximizing expected utility. Finally, nonrational models are often more likely to emphasize the role of social and context-specific factors in decision making.
Each of these features of nonrational models can be elaborated upon in turn. First, nonrational models of decision making give emotions and other non-cognitive factors a greater role than rational models do. It is well-established that emotions can play a significant role in decision making (for better or for worse). Nonrational models of decision making take this into account and give emotions a greater role in the decision making process. This is not to say that emotions are the only factor that nonrational models take into account, but they are certainly given a greater role than in rational models.
Second, nonrational models de-emphasize or even ignore the role of deliberative reasoning in favor of more intuitive processes. This is a significant departure from rational models, which give a great deal of weight to deliberative reasoning. Nonrational models may favor more intuitive processes for a number of reasons. First, intuitive processes may simply be more efficient than deliberative reasoning. Second, intuitive processes may be more likely to lead to the correct decision, or at least a decision that is more satisfactory to the decision maker. Finally, intuitive processes may simply feel more natural to the decision maker and be more comfortable to use.
Third, nonrational models often involve a greater degree of risk taking and are less concerned with the idea of maximizing expected utility. This is again a significant departure from rational models, which tend to be quite risk-averse. Nonrational models may be more willing to take risks for a number of reasons. First, nonrational models may place a greater importance on the possibility of a "big win" than on the more likely "small losses." Second, nonrational models may be more concerned with the immediate consequences of a decision than with the long-term consequences. Finally, nonrational models may simply be more comfortable with taking risks than rational models are.
Finally,
You might like: Kia Models
How do nonrational models differ from rational models?
Nonrational models are those which do not attempt to accurately capture and predict human behavior. They are usually much simpler than rational models, and they are often based on heuristics or rules of thumb. Nonrational models often make assumptions about human behavior that are not always supported by empirical evidence. As a result, nonrational models are often less accurate than rational models.
One of the key differences between nonrational and rational models is the way in which they handle uncertainty. Rational models typically assume that humans are rational actors who make decisions based on a clear understanding of the situation and the likely outcomes. Nonrational models, on the other hand, allow for the possibility that humans may act irrationally or make decisions based on emotions or other non-rational factors.
Another key difference between nonrational and rational models is the way in which they deal with risk. Rational models typically assume that humans are risk-averse and will make decisions that minimize potential losses. Nonrational models, however, may allow for the possibility that humans may take risks in order to achieve a desired goal.
Nonrational models often have more limited applications than rational models. This is because they usually make simplifying assumptions about human behavior that are not always accurate. For instance, nonrational models of consumer choice often assume that all consumers are the same and that they all make decisions based on the same factors. However, we know that consumers are individuals with different needs, preferences, and constraints. As a result, a nonrational model of consumer choice is likely to be less accurate than a rational model.
Overall, nonrational models differ from rational models in a number of ways. They are usually simpler and based on heuristics or rules of thumb. They often make assumptions about human behavior that are not always supported by empirical evidence. As a result, nonrational models are often less accurate than rational models.
Explore further: Which of the following Is Not a Characteristic of Metals?
What are some common criticisms of nonrational models?
Nonrational models are criticized for a number of reasons. One common criticism is that they are too simplistic and do not take into account the complexities of human behavior. Additionally, nonrational models are often based on outdated ideas about human cognition and decision-making, which can lead to inaccurate predictions. Additionally, nonrational models often ignore important contextual factors that can influence decision-making, such as emotion and social pressures. Finally, nonrational models can be difficult to generalize to real-world situations.
For more insights, see: Pimco Models
How do nonrational models account for bounded rationality?
Bounded rationality is a term coined by decision-making researcher Herbert A. Simon. It basically means that humans are not rational beings. We do not always make the best decisions because we are limited by our cognitive abilities, time, and information. We are also influenced by our emotions and biases.
Simon argued that because of these limitations, we often satisfice instead of optimize. Satisficing is when we choose the first option that meets our requirements, even if there are better options available. For example, when we are looking for a new car, we might not compare all of the different makes and models before choosing one. We might just pick the first one that meets our needs.
Simon’s Theory of Bounded Rationality has been used to explain a lot of human behavior. For example, it has been used to explain why people procrastinate, why we have a hard time making decisions, and why we are often bad at predicting what we will want in the future.
There are many different models of bounded rationality. Some of the most popular ones are the Elaboration Likelihood Model, the Heuristics and Biases Approach, and the Satisficing Model.
The Elaboration Likelihood Model was developed by social psychologists Richard Petty and John Cacioppo. It suggests that there are two different ways that we process information: the central route and the peripheral route.
The central route is when we process information deeply and thoughtfully. We pay attention to the arguments and evidence presented. We weigh the pros and cons. We come to a reasoned conclusion.
The peripheral route is when we take shortcuts in our thinking. We rely on heuristics, or mental shortcuts, to come to a conclusion. We don’t pay attention to the arguments and evidence. We might just go with our gut feeling or choose the option that is the least effortful.
The Heuristics and Biases Approach is a bit different from the Elaboration Likelihood Model. It doesn’t focus on the two different routes of information processing. Instead, it focuses on the biases that can influence our decision-making.
Some of the most common biases that can distort our judgment are confirmation bias, sunk cost fallacy, and theavailability heuristic.
Confirmation bias is when we pay more attention to evidence that supports our beliefs and less attention to evidence that contradicts our beliefs. For example,
Additional reading: Final Decision
How do nonrational models account for emotions and other psychological factors?
There are many nonrational models that can be used to account for emotions and other psychological factors. One popular model is the Josephson model, which posits that emotions are based on irrational and illogical thought processes. This model has been found to be particularly accurate in predicting how people will react in different situations. Another nonrational model, the Oatley-Johnson model, focuses on the role of emotions in decision-making. This model argues that emotions play a significant role in our ability to make decisions, and that we often base our decisions on our emotional state. This model has been found to be particularly accurate in predicting how people will make decisions in ambiguous situations.
Consider reading: Nims Structure Makes Cooperative Multi Agency Decisions
What are some common heuristics used in nonrational models?
There are a variety of heuristics that are commonly used in nonrational models. Some common examples include the following:
1) The law of small numbers: This heuristic suggests that people tend to underestimate the variability of small samples and overestimate the variability of large samples. This can lead to errors in judgment when making decisions based on limited data.
2) The representativeness heuristic: This heuristic suggests that people often judge the likelihood of an event occurring by how well it represents the available information. This can lead to errors in judgment when the available information is not representative of the true underlying distribution.
3) The availability heuristic: This heuristic suggests that people often judge the likelihood of an event occurring by how easily it comes to mind. This can lead to errors in judgment when the availability of information is biased or distorted.
4) The anchoring heuristic: This heuristic suggests that people often rely too heavily on the first piece of information they receive when making decisions. This can lead to errors in judgment if the initial information is not accurate or representative of the true situation.
5) The sunk cost fallacy: This heuristic suggests that people often consider sunk costs when making decisions, even though these costs should not impact the decision. This can lead to bad decision-making if the sunk costs are not taken into account properly.
6) The gambler's fallacy: This heuristic suggests that people often believe that past events can influence future events, even when there is no rational basis for this belief. This can lead to errors in judgment when making decisions based on random chance.
7) The confirmation bias: This heuristic suggests that people often seek out information that confirms their existing beliefs, while ignoring information that contradicts their beliefs. This can lead to a biased view of the world and errors in judgment.
Worth a look: When Making Others Happy Is Making You Miserable?
How do nonrational models deal with uncertainty?
Nonrational models are mathematical models that do not rely on the assumption of rationality. This means that they are not based on the idea that people always make rational decisions. Instead, nonrational models account for the fact that people sometimes make irrational decisions.
There are a variety of ways to deal with uncertainty in nonrational models. One approach is to use a Monte Carlo simulation. This technique involves repeatedly generating random numbers and using them to generate different possible outcomes. The probabilities of each outcome are then estimated based on the number of times that outcome occurs.
Another approach is to use a series of decision trees. This approach involves making a series of decisions, each of which has a certain probability of leading to a successful outcome. The probabilities of each decision are then multiplied to get the overall probability of success.
Yet another approach is to use a genetic algorithm. This approach involves creating a population of potential solutions and then using Evolutionary algorithms to find the best solution. The Genetic algorithm is a type of Evolutionary algorithm that is particularly well suited to dealing with uncertainty.
All of these approaches have their advantages and disadvantages. Which approach is best depends on the specific problem that is being tackled.
For another approach, see: Which Is Not a Characteristic of Mitochondria?
What are some common applications of nonrational models?
Nonrational models are mathematical models that are not based on traditional assumptions of rationality. Some common applications of nonrational models include games and decision-making.
Games
Nonrational models are often used in game theory to help analyze situations where players may not be fully rational. For example, a nonrational model might be used to analyze a situation where two people are trying to decide how to divide a piece of cake. In this situation, each person may not be fully rational and may instead try to maximize their own share of the cake. By using a nonrational model, game theorists can better understand how people might behave in such a situation.
Decision-making
Nonrational models can also be used to help people make decisions. For example, a nonrational model might be used to help someone choose between two jobs. In this situation, the person may not be fully rational and may instead try to maximize their own utility. By using a nonrational model, the person can better understand how they should make their decision.
Additional reading: Equity Valuation Models
What are some limitations of nonrational models?
There are limitations to nonrational models, which include the following:
1) They cannot fully explain human behavior.
2) They may produce inaccurate predictions.
3) They may be oversimplified.
Frequently Asked Questions
What are the various types of non-rational decision making models?
1 Bounded Rationality:#N#ADVERTISEMENTS:#N#This, in fact, is a non-rational model. In such case, the individual decision maker operates within certain preset boundaries set by rationality. Such a model might be appropriate when the decision needs to be made quickly or when there is limited information available. 2 Incremental Model:#N#Decisions using such model are essentially short term, and therefore, lack long-term perspective. This might be suitable for decisions that need to be taken near-immediately but may not have lasting ramifications. 3 Garbage Can Model: More ...
What is non rational decision making theory?
A nonrational theory is a decision making model that focuses on problems with traditional "rational" models. Nonrational theories are designed to overcome the limitations of these models. There are three main types of nonrational theories: models of bounded rationality, procedural rationality, and satisfying.
How to differentiate between rational and non-rational models of decision-making?
The answer to this question can be found in the following distinctions: 1. Rational models are normative. They prescribe how things ought to be, rather than what is objectively true. For example, when it comes to personal finance, most people adhere to a rational model that prescribes specific financial goals and strategies. 2. Rational models are theoretical or unrealistic in nature. They may be based on idealized assumptions about human behavior and organizations. Because they are not grounded in empirical reality, they can be difficult to apply in real-world situations. 3. Rational models follow deterministic approach to problem-solving. They believe that there exists an optimum solution for any given problem, and that all information needed to reach that solution is already available. This approach can be limiting because it does not take into account potential surprises or unforeseen circumstances. 4. Rational models believe there exists the optimum situation for decision-making. This means that decision-makers focus on finding the
When do teams use the rational decision model?
Typically, teams use the rational decision model when they have time for meetings and research.
What are the different types of decision models?
There are three main types of decision models - rational models, intuitive models, and rational-iterative models. Rational models try to bas their conclusions on cold, hard facts, while intuitive models try to take into account people's feelings and assumptions. Rational-iterative models combine elements of both intuition and rationality by allowing for feedback between the various stages in the model.
Sources
- https://www.studymode.com/essays/Two-Nonrational-Models-Of-Decision-Making-68297851.html
- https://quizlet.com/ca/487446372/management-110-part-2-flash-cards/
- https://quizlet.com/531915998/management-chapter-7-flash-cards/
- https://www.ipl.org/essay/Nonrational-Model-Of-Decision-Making-P3KZ86HE28VT
- http://www.nationalforum.com/Electronic%20Journal%20Volumes/Lunenburg,%20Fred%20C%20Models%20of%20Decision%20Making%20Focus%20V4%20N1%202010.pdf
- https://www.coursehero.com/study-guides/boundless-management/rational-and-nonrational-decision-making/
- https://www.sciencedirect.com/topics/engineering/rational-model
- https://www.modeltheory.org/about/critics/
- https://pubmed.ncbi.nlm.nih.gov/21875170/
- https://link.springer.com/chapter/10.1007/978-3-319-60535-7_5
- https://pvillage.org/archives/134406
- https://www.chegg.com/homework-help/questions-and-answers/200-words-two-models-nonrational-decision-making-q24161221
- https://plato.stanford.edu/entries/bounded-rationality/
- https://study.com/learn/lesson/bounded-rationality-theory-model.html
- https://web.stanford.edu/~icard/Lecture1-h.pdf
- https://www.researchgate.net/publication/2937619_Bounded_Rationality_Bounded_Models
- https://www.researchgate.net/publication/225967899_Bounded_Rationality_Modeling
- https://www.researchgate.net/profile/Yesim-Korkut/publication/338795322_Integrating_emotion_and_other_nonrational_factors_into_ethics_education_and_training_in_professional_psychology/links/609293b1299bf1ad8d7b2f51/Integrating-emotion-and-other-nonrational-factors-into-ethics-education-and-training-in-professional-psychology.pdf
- https://www.aucccd.org/assets/rogerson%20-%20ap%20-%202011.pdf
- https://link.springer.com/referenceworkentry/10.1007%2F978-1-4419-1428-6_728
- https://corporatefinanceinstitute.com/resources/knowledge/other/heuristics/
- https://clikfocus.com/blog/12-types-of-heuristics
- http://alae.alfa145.com/what-are-heuristics-how-heuristics-are-used-in-problem-solving/
- https://www.reddit.com/r/MachineLearning/comments/70j9dg/d_what_are_some_common_heuristics_used_in_rnn/
- https://stackoverflow.com/questions/53916514/what-are-some-heuristics-for-structuring-scalable-model-relationships-in-django
- https://hbr.org/2020/08/how-to-make-rational-decisions-in-the-face-of-uncertainty
- https://towardsdatascience.com/model-averaging-a-robust-way-to-deal-with-model-uncertainty-a604c4ab2050
- https://sustainability-innovation.asu.edu/docs/dcdc/website/documents/DaveWhite_DCDCWaterEducatorsWorkshop_Final.pdf
- https://d3dqsm2futmewz.cloudfront.net/docs/dcdc/website/documents/DaveWhite_DCDCWaterEducatorsWorkshop_Final.pdf
- https://link.springer.com/chapter/10.1007/978-1-4612-3412-8_6
- https://serc.carleton.edu/teachearth/teaching_methods/models/limits.html
Featured Images: pexels.com